The difference between 67% and 80% will shock you.
For modern contact centers aiming to understand customer interactions at a deeper level, transcription accuracy is where it all starts. To nail accuracy, companies with best-in-class automatic speech recognition (ASR) engines use AI services to not only understand what is being said on a call, but how it is being said by assessing tonality, silence, pauses, and so much more.
That means getting transcription right is mission-critical. When transcription is inaccurate, processes break down. And for the modern contact center, that means you can’t pave the way for a future powered by automation. Why?
- You cannot trust the speech analytics insights derived from calls to make sense of your top-level contact center KPIs and agent performance.
- You can’t fairly and effectively score performance. Disputes arise, and agents won’t trust the feedback they’re receiving. Unfortunately, some businesses are making lay-off and termination decisions off of really, really inaccurate data.
- You can’t build impactful and personalized coaching programs. Leveraging transcripts to coach agents with context is impactful, but not if a bunch of translation errors and misspellings prevent them from even knowing what happened in the conversation.
Accurate transcription isn’t a nice to have. It’s a requirement - and the better your transcription, the better your insights, agent enablement, and ROI.
But don’t just take our word for it. Here’s how it actually works in the real world.
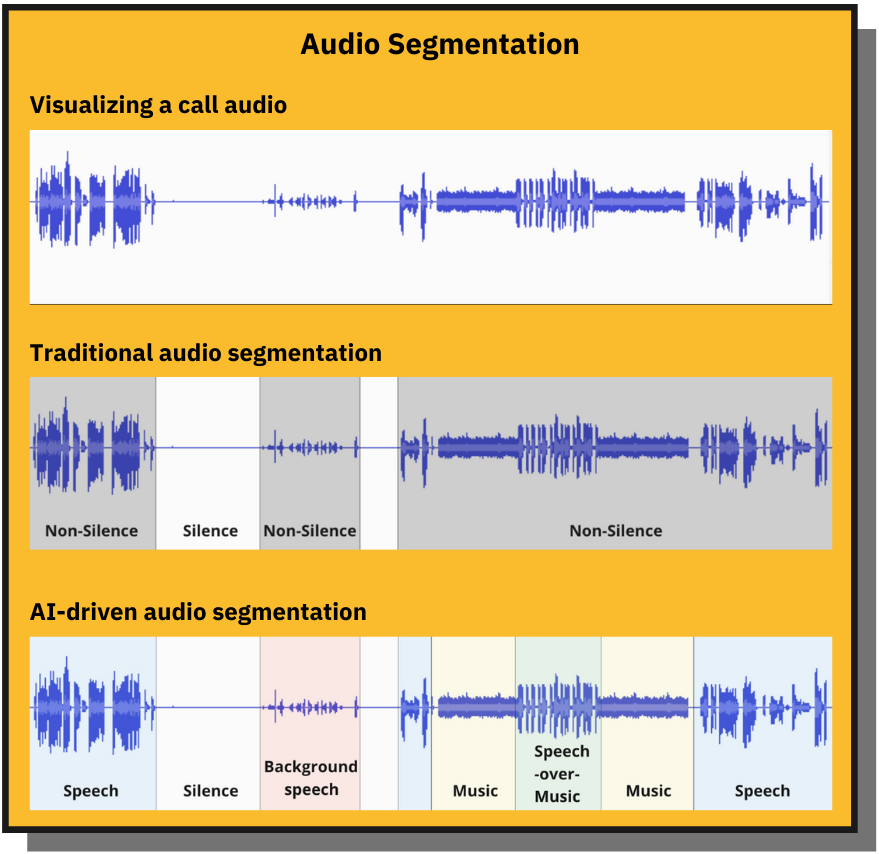
Transcription accuracy: every % matters
“If the accuracy piece isn’t there, the other bells and whistles don’t matter.”
- one of our Fortune 500 customers who switched over from a legacy speech analytics solution
“It looked like someone put words in a blender.”
- another one of our customers who evaluated three other solutions
The difference between 67% and 80% transcription accuracy is shocking. In the diagram below, you can see the actual transcript, and two transcripts at varying percentages. At 67%, it’s virtually useless. At 80%, there are mistakes, but the content of the transcript is understandable.
The 13% difference in accuracy makes or breaks the transcript.

What is transcription? Core tenets to look for
Transcription is the process of converting audio into a text transcript. In a contact center, this transcript is primarily a call between a customer service agent and a customer.
AI services have long changed the transcription game. With the emergence of automatic speech recognition (ASR), voice calls can now be automatically transcribed at a massive scale in real-time very soon after they’re completed.
From there, a number of natural language processing (NLP) services are used to analyze and derive insights from the transcript and its accompanying audio file. NLP understands language, draws patterns, derives trends, categorizes, groups, and structures unstructured data. This is where those valuable insights - such as insights on customer sentiment, dead air, and hold time - emerge.
Transcription accuracy benchmark
The industry benchmark for transcription (defined by Google and Amazon), is 72%. We’ll keep ours at 80% minimum, thanks, but strive for higher.
Transcription challenges: why it’s hard to get right
ASR is a machine learning-based system. That means that it only works well when transcribing the kind of data that it’s already seen and trained itself on. New words, phrases, accents, dialects, call types, call quality, and more can throw a wrench in the system, and as a result, impact the transcription accuracy.
A good ASR service gets smarter over time. As an example, we’ve trained our proprietary ASR engine on more than 10 million contact center calls. Our engine was built to specifically recognize and take on the challenges of customer service conversations and can mitigate things like over talk, make sense of conversations taking place over far distances, recognize hold time music, and more.
Beyond machine learning capabilities, accounting for tonality is equally complex. The transcript shouldn’t just determine what was said, but analyze how it was said as well. That includes understanding pitch, volume, and tone.
And lastly, accurate transcription is driven by industry context. An ASR service built for a specific purpose is going to outperform a general ASR service every time. Not all ASR services are built equal.
For example, Alexa and Google Assistant are built for consumers. They excel at turning on lights, changing songs, and adding groceries to a list. That same technology cannot be applied to contact center call analysis. It’s an entirely different use case, and as a result, the service is built and trained differently.
What are the building blocks of a world-class ASR?
A contact center AI platform with an ASR engine that accounts for the challenges listed above delivers higher value, more accurate insights, and in turn, is the basis for future workflow and process automation. When you’re evaluating contact center AI solutions, ask yourself these questions to get a detailed picture of accuracy.
Does the ASR take industry into account?
It’s simple. An ASR built for contact center conversations is better than a broad ASR. Why? Building ASR on a narrow data set based on contact center calls boosts the accuracy of the relevant keywords resulting in higher transcription accuracies. An engine trained on financial services-related customer service will better understand and transcribe the intricacies of those conversations.
Examples of this include phonetic matching, adding correct customer names or product names, which are not in standard ASR vocabulary. Think words like Peloton, Observe.AI, and T-Mobile. Beyond what is being said, it’s equally important to account for the qualities of the speaker, and using custom models, the ASR can account for accents, dialects, and customer-specific vocabulary.
Does the ASR train itself and consistently improve?
Transcription self-learning and tuning is critical, especially for customer-specific words and phrases, and plays a huge impact on the business use cases for a contact center. In the broadest sense, a self-learning ASR engine makes predictions on two categories - common and customer-specific words.
The major differentiator when it comes to accuracy is driven by approaches to language model corrections. At Observe.AI, we call our award-winning post-processing engine Korekti, because it corrects common words with customer-specific words - things like cash --> cache, trend --> tread, like --> bike.

Can the ASR accurately identify different speakers?
What good is a call transcript if it doesn’t accurately show who is saying what in the conversation?
This is especially crucial for insights gathering. Is it the agent or the caller who is using a negative tone? Who is committing the compliance violation? To get accurate insights, you need confidence that the transcription service can decipher different speakers and structure the call transcript accordingly.
The results: Big potential, bigger benefits
At the end of the day, the more accurate your transcription, the better the results you’ll get - from more impactful coaching programs, to stronger compliance mitigation, to more efficient QM automation. It all starts with the quality of the call transcript. Let’s look at a couple game changing opportunities accurate transcripts unlock for you.
Deep sentiment analysis
The complexity of the spoken word makes gauging sentiment incredibly challenging. Different words, phrases, tone, volume, accents, and dialects are all considered when analyzing customer conversation with sentiment analysis.
Take empathy statements as an example, which are powerful phrases that agents can use to build better rapport and connect with customers. Marrying transcripts with sentiment analysis, teams can see which empathy statements drove KPIs like growth per call, CSAT, and Net Promoter Score (NPS) -- helping teams make sense of not just what was said, but how it was received. These statements can then be used in future coaching sessions, such as trainings on how top agents turned customers into raving fans.
Compliance and fraud detection
Contact centers handle massive amounts of sensitive information on a daily basis. Compliance violations can lead to damaged reputation, loss of revenue, and stiff penalties. Compliance mitigation relies heavily on accurate transcription for a couple reasons.
- Detecting and redacting PII: redaction rests hand-in-hand with the call transcript. The transcription service must be able to identify PII so it can be redacted. Today, top-notch speech recognition systems will enable Selective Redaction. This is where parts of an entity are redacted, while remaining portions are still visible. This solves the issue of over-redaction or under-redaction, and allows contact centers to customize automated redaction to their unique needs. For example, some organizations might want to redact a social security number, but leave a birthday visible.
- Monitoring for mandatory compliance dialogues: for teams where mandatory compliance dialogues need to be stated on every call, those statements must be recognized and reported on. Agents who miss them must be identified and coached. Accurate transcripts unlock opportunities for compliance leaders and supervisors to be alerted when risks happen in near real-time before they spiral out of control.
- Creating a paper trail: in the event of an audit, call transcripts are an audit trail that can be used to prove compliance. Imagine holding up a terribly inaccurate transcript in court!
The result is stronger compliance mitigation, and in turn, your CCO sleeps easy at night.
Personalized coaching with context
The most successful agent coaching programs are those that coach with context, where real examples reinforce the coaching sessions.
Transcripts are the basis of powerful coaching content. Take conversation snippets for example. Snippets are an effective way to up the value of a QA evaluation form, where QA analysts can reference an exact moment on the call and provide clear feedback on how to improve it.
And what about disputes? An accurate transcript puts that to bed, delivering a single source of truth. Agents can see their own call recordings, transcripts, and performance reports, minimizing disputes, and in turn, reducing significant workload for QA analysts.
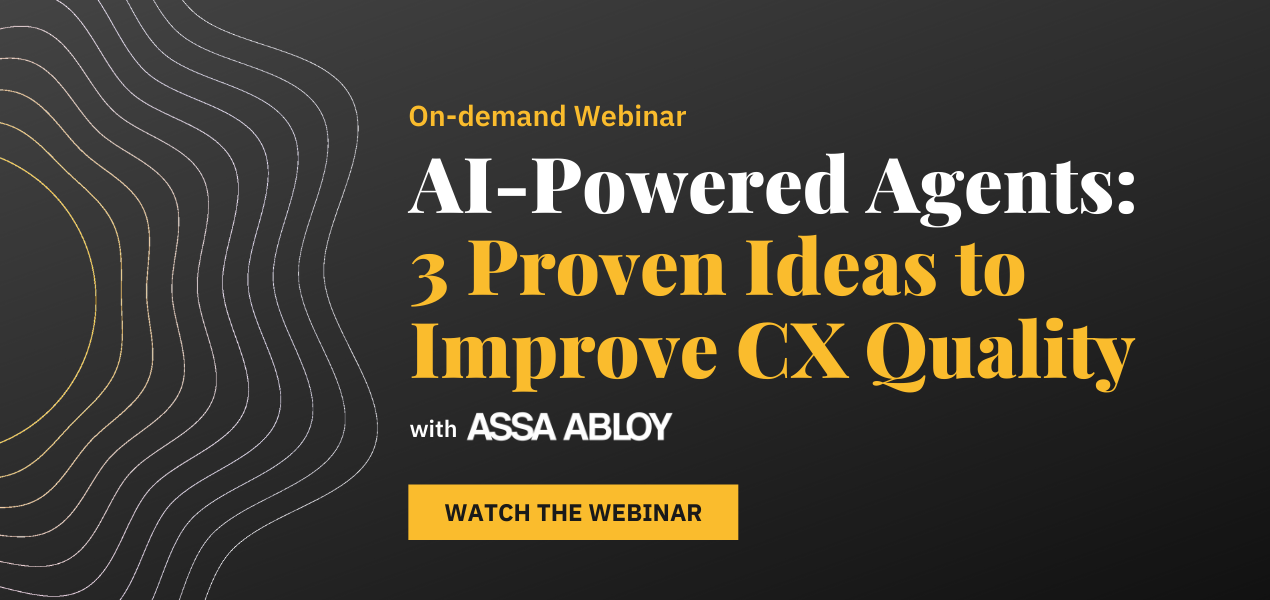
Paving the future for better automation
As with any platform or technology built on AI-services, the promise is always faster, more accurate, and more efficient automation.
Like all contact center leaders, you’re probably wondering, how can we streamline processes to make our business more resilient? How can we extract more valuable data from unknown sources? How can we deliver value to everyone at the contact center - from the agents on the frontlines to leadership?
Accurate transcription is a crucial piece of that puzzle. After all, all the analysis takes place on that transcript. All the automation stems from that transcript, too.
Thus, investing in a platform that invests heavily in their ASR engine, and has built it for a specific data set (the contact center), will yield better insights, agent enablement programs, and ultimately ROI.
