Deeply understanding the customer experience has been a black box for decades.
As a result of COVID-19, consumer behavior has shifted considerably with 73% of consumers changing stores, brands and the ways they shop, forcing organizations to re-think how the customer experience (CX) is measured, quantifying the black box.
Thanks to continued advancements in technologies like Artificial Intelligence (AI), speech-to-text, and Natural Language Processing (NLP), organizations are finding that AI-powered CX analytics can increase conversions by up to 50%.
With organizations planning to maintain complete or hybrid teams, the urgency to use AI has increased dramatically— mainly to get better visibility into agent performance, customer sentiment, and brand perception.
A key piece in delivering delightful, personalized, and differentiated customer experiences, particularly for the voice channel, is sentiment analysis.
In this blog, we’ll explore:
- How AI helps businesses quantify voice insights
- How it gains context behind customer interactions
- And different ways in which next-gen contact centers are delivering exceptional customer experiences using these insights
What is sentiment analysis?
Sentiment analysis is a machine learning application that sheds light on the opinion of a speaker towards a product, person, topic, or event.
It’s applied by allowing organizations to ingest and analyze millions of recorded audio calls using speech recognition and Natural Language Processing (NLP). This allows businesses to analyze customer and agent interactions on a deeper level like automatically detecting positive, negative, or neutral interactions on calls.
In turn, customer experience teams can identify unmet customers needs and new opportunities to improve service quality.
To help explain the concept of sentiment analysis, we’ll use one of our favorite analogies:
Universally, we’ve all heard our partners say, “Honey, I’m home.” Often, you can easily identify the mood of your partner’s voice, thanks to the innate human understanding of tone and inflection.
Now imagine just reading the words— “Honey, I'm home.”
With no context, one will automatically assume things are okay.

we can easily identify a person's sentiments.
Rather than perfectly interpreting human emotions on its own, AI can assist quality analysts and business intelligence teams in drilling down on customer interactions that require further analysis.
It helps in narrowing down the scope of call investigations and answers questions like— was this a one-off negative experience? Is it a quantifiable trend?
This is called a “Human in the loop” approach, where people can leverage AI to increase efficiency and precision to assist in repetitive work, such as manually listening and transcribing voice calls.

How does sentiment analysis work?
When we first launched our platform in 2017, we built a proprietary Automatic Speech Recognition (ASR) engine.
It has since been trained on over 10 million+ hours of customer service calls.
Our ASR engine automatically transcribes, analyzes, and evaluates quality assurance on 100% of a business’s customer service calls.
With its tonality-based sentiment detection, our ASR engine is capable of not only understanding what is said on a call, but how a customer is saying it.
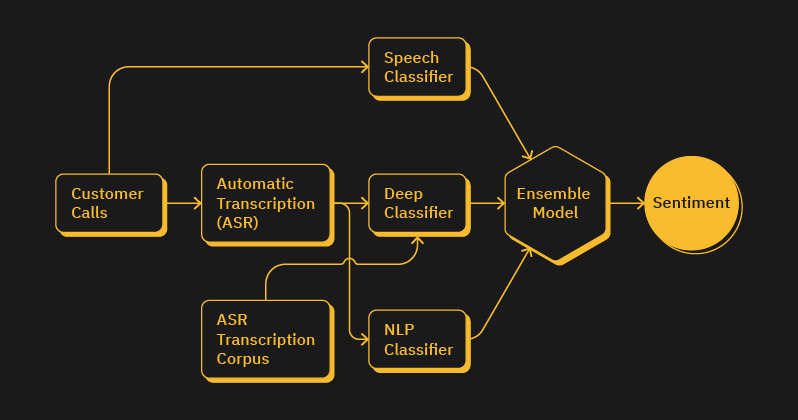
Our ASR engine identifies patterns in areas like tone, speech rate, and speaker volume that are then superimposed over text to meaningfully understand the context of the conversation.
With our speaker diarization system, we also split an input audio stream into homogeneous segments to accurately identify who is speaking.
The platform then unlocks customer sentiment to fuel smarter, faster agent evaluations by allowing quality teams to access points-of-interest in the transcript right alongside an audio player and agent evaluation form.
Let’s take a look at some of the top use cases of how businesses use sentiment analysis to improve the overall customer experience.
3 Powerful Ways Sentiment Analysis Can Assist Businesses

1. Uncover unmet customer needs
In today’s highly competitive market, the best business growth opportunities can be found deeply embedded in a customer's unmet needs and expectations (driven through speech analytics).
Here’s a great sentiment analysis example of the automobile industry successfully met their customer needs.
One high growth auto repair startup was able to evaluate 100% of its calls, and find key moments where customer frustration was peaking. They quickly learned that the most frequent peaking was when a customer’s zip code was not yet being serviced.
On further investigation, they identified the zip codes and products that were needed to better meet customer expectations. This enabled them to bring a proposal to the management that clearly highlighted hundreds of thousands of dollars in untapped revenue
2. Inform feedback on products and services
The voice channel continues to be the #1 channel to offer the most in-depth insights. A recent Zendesk survey found that phone is still the most used (48%) and most preferred (80%) method for resolving customer service queries.
This logistics company evolved their entire customer service experience with just a tweak.
JK Moving Services, a national moving company, used sentiment analysis to determine if there were gaps in their products or services during COVID.
When looking into mentions of COVID in parallel to instances of positive or negative customer sentiment, JK Moving found something rather surprising.
Customer service reps who proactively mentioned safety protocols, such as
- “the movers would show up in face masks”
- “The movers have gone through a rigorous safety training specific to moving during COVID”

Keywords and phrases can be tracked on the platform to understand customer needs
These phrases led to happier and assured customers. Needless to say, these proactive reps eventually went on to close more deals—and bigger ones, too. In response, the company updated their scripts to ensure customers were informed of safety protocols at every step of the process to scale the best practices used by top reps.
A simple solution to the biggest threat we are facing today.
“Having Observe.AI be an extra “set of eyes and ears” has been invaluable.”
—Ken Cohen, JK Moving.
3. Elevate the employee experience
Organizations aren’t just using sentiment analysis to understand the health of their customer experience. They’re also leveraging sentiment analysis to better gauge their employee experience.
With the sudden shift to remote work, organizational leaders are looking for new ways to ensure their remote teams are safe, motivated, and well-supported.

At Emtek Assa Abloy, the quality assurance (QA) team is able to leverage the Observe.AI platform to help coach agents and better respond to their needs.
Observe.AI allows their QA team to understand and quickly address logistical issues faced by their agents like network connectivity, head-set issues, uncontrollable background noise, etc.
They’re also able to narrow down agents who need more help and better support via personalized coaching.
Another example of the benefits of sentiment analysis is of a pharma e-commerce company. One of their agents working remotely wasn’t able to handle an irate, disrespectful customer. Later, the supervisor could easily view the interaction via the Observe.AI leaderboard, and provide necessary tips on how to handle such customers.
Final Words.
For decades, sentiment analysis has been vaguely measurable due to limited or unstructured data, leaving the nuances of customer success to an individual agent’s skillset. AI and Natural Language Processing (NLP) have together unlocked a way to scale and identify what triggers positive or negative brand perception, giving us a glimpse into what was otherwise undetectable. As we look into the future, leveraging tonality-based sentiment analysis to revamp business processes rapidly will redefine what it means to be truly customer-centric.

Check out some of these helpful resources:
Ebook: The Seven Most Critical Soft Skills for Agents
Webinar: Driving Disruption with AI: CX Experts Panel
Blog: What are Empathy Statements, and how do you coach agents on them?