You may be tired of all the Generative AI and ChatGPT talk already. But that doesn’t mean you should ignore it.
Whether we like it or not, it’s here to stay—and your competitors are likely experimenting with it already.
However, there’s one major mistake they may not be aware of: Most LLMs are not suitable for contact center operations.
We explain why below and share the results of several benchmarking tests we ran against GPT 3.5 to prove it.
Why Contact Center-Specific LLMs Matter
At Observe.AI, we have been using large language models (LLMs) since the time they came into forefront, starting with the famous BERT model (for the last 5 years). However, unlike the traditional method of fine-tuning the out-of-box language models, we trained our own models to learn the characteristics of contact center data.
The out-of-box language models (LMs) are trained on clean text corpuses, such as articles from Wikipedia or other stories that have been through a rigorous editing and proofreading process.
For these LLMs, it is a major challenge to analyze and decipher noisy and out-of-domain data, like contact center conversations, where automatic speech recognition (ASR) errors are prevalent in the transcribed text.
In addition, natural conversation between agents and customers can be especially confusing due to disfluencies (stuttering or the stopping and starting of sentences), repetition of information, or non-grammatical utterances..
We built LLMs customized for contact center data and trained them to perform well for contact center use cases:
- Automatic summarization of conversations
- Generation of coaching notes
- Helping agents query knowledge bases
- Extracting various insights, like sentiment analysis and reasons for a call
We trained models with different sizes: 7B, 13B, 20B, and 30B parameters to get maximum performance for individual tasks.
Benchmarking Against GPT 3.5
We benchmarked our proprietary LLM with GPT3.5 models to prove a contact center-specific LLM would be more accurate and effective.
Let’s start with the task of call summarization.
When asking the machine to summarize a call, we’re not just asking it to give us a high-level abstract of what took place. There are specific elements that need to be interpreted and identified:
- Reason for the call
- Important events and actions that took place
- Opportunities presented by the agent
To evaluate the performance objectively, we conducted a comparative analysis between our in-house contact center LLM and GPT3.5 on these attributes.
In the blind evaluation, we prompted both platforms to identify the call reason, resolution steps, and sequence of events using real-world customer conversations (after all sensitive entities had been redacted).
We then had human evaluators review the data (without being told which was our LLM or GPT3.5) and determine if the output was acceptable.
Our findings indicate that our in-house LLM demonstrates a higher accuracy in identifying and generating the key actions and events within the call, including the relevant entities discussed.
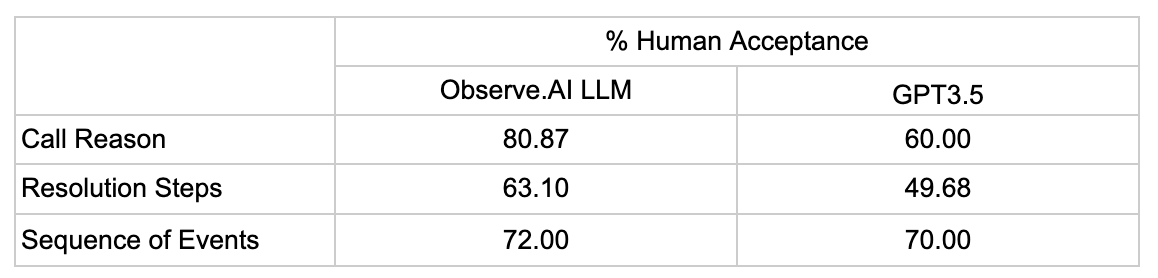
The table above shows the percentage the evaluators considered the output to be accurate. So for example, when evaluators analyzed the results for “Call Reason” they found 80% of the outputs from Observe.AI’s LLM to be accurate, while GPT3.5 was only accurate 60% of the time.
When it comes to generating next steps, we observed that GPT3.5 has a tendency to produce logical yet hallucinated suggestions, in contrast to our in-house model.
Notably, both models perform comparably in detecting the sequence of events.
We continuously build newer LLMs which will help us not only to customize them to contact center data but also leverage the new generative AI techniques to enable more AI use-cases.
Interested in learning more about how our contact center LLM can help drive better performance and efficiency in your contact center? Schedule a meeting with one of our experts.